The Need for Trustworthy Artificial Intelligence
And the importance of asking the right questions
Modern artificial intelligence is transforming the 21st century. A phenomenal worldwide effort aims to unlock the benefits of AI, across society, business, and the economy. These include the automation and scaling of manual tasks as well as the discovery of highly efficient, accurate, and faster solutions, some of which can be described as super-human solutions to age-old problems.
Yet embedding AI in society presents a complex mix of technical and social challenges, not the least of which is: as more decisions are delegated to machines that we cannot fully verify, understand, or control, will AI systems be trusted?
This is a question that seems to be being asked a lot, of late. And unfortunately, I don’t think we are near any sort of satisfactory answer. In my opinion, we don’t even understand the question properly, yet.
For a start, trust decisions are always situational. As my friend and colleague at Ontario Tech, trust scientist Steve Marsh likes to say: ‘I might trust my brother to drive me to the airport, but I’m not going to trust him to fly the plane!’
Unfortunately, this is a factor that is all to often lost in the AI space: see as one example of many, this Twitter poll that simply asked ‘do you trust #AI?’.
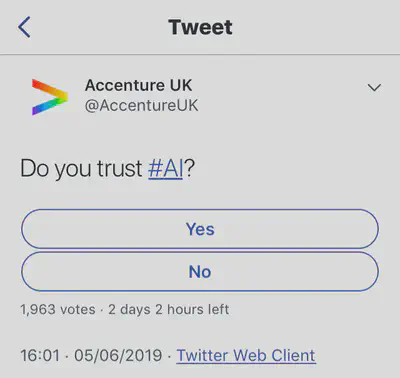
Whilst it is definitely possible to have some foundational level of trust in specific others, trust is inherently contextual. Asking if you trust AI is akin to asking if you trust the human race: perhaps a nice headline, but in any event, meaningless.
And, even if we answer ’no’ what does that actually mean, given the situation we are already in?
My approach is to work towards helping people to make good trust decisions about intelligent machines of different sorts, in different contexts.
How can we conceive of and build intelligent machines that support people to do this well?
The Urgency
Understanding and enhancing the trustworthiness of AI are pressing needs. And it is easy to see why: Microsoft’s Tay, the chatbot capable of learning, turned racist; Amazon’s automated recruiting tool, trained on data from previous hiring decisions, discriminated based on gender for technical jobs; intelligent scheduling tools are oblivious to important and obvious human values, to the detriment of workers; and the racist nature of facial recognition technology is so accepted that black candidates taking the Bar Exam in the United States are being advised to do so with a bright light shining on their face. And these are just the examples that made the news.
In response, statements concerning the principled and ethical use of AI are being developed, both company-specific, such as SAP’s Guiding Principles for Artificial Intelligence, and for society at large, such as the Asilomar AI Principles.
In 2019, the EU published its Ethics Guidelines for Trustworthy AI. There’s a lot to unpack here and the documents that followed. But to summarize, in its view, trustworthy AI will be:
Lawful, respecting all applicable laws and regulations;
Ethical, respecting ethical principles and values; and
Robust, both from a technical perspective and while taking into account its social environment.
But, as important as these initiatives are (and they are!), what does this mean? There seems to be the implication here that guidelines are telling us how AI should be built or behave in order to be seen as ’trustworthy’. Presumably this means that people are going to (should? must?) trust it, if we the engineers follow along.
In Canada, Deloitte’s report Canada’s AI imperative: Overcoming Risks, Building Trust notes that poor understanding of AI is contributing to ‘a rising distrust of AI among businesses, governments, and citizens alike’, and argues that this could have serious negative impact on Canada’s prosperity and position as a beacon of AI R&D. It further argues that it is people’s perceptions of AI that will drive trust, or lack thereof.
These initiatives are vital in supporting governance of AI, yet are the start of an answer to our question.
Value alignment has focused on capturing universal human values in AI systems. However, trust decisions are personal, human norms and values often depend on socio-cultural context, and interpretation of behaviour varies. Consider differences in the use of tone of voice; attitudes to touching and personal space (and how quickly this can change in the presence of a pandemic); whether to kiss, shake hands, bow, or bump elbows upon meeting; and when one should give up a seat on the bus.
Indeed, the IEEE’s Ethically Aligned Design report argues that it is essential that intelligent systems ‘are designed to adopt, learn, and follow the norms and values of the community they serve.’
How should we build intelligent machines to do that?
Trust Empowerment
There will be people who will respond to this with a call for full verification and certification before a particular AI technology is ‘released’. But a bit of reflection on this proposal quickly reveals this to be well-intentioned but inadequate.
As AI systems grow in complexity, I believe we are faced with a choice. Either:
Remove the need for trust by removing the risk, either by limiting the use of current and future AI to only low-risk cases, or narrow domains where full verifiability (including any humans in the loop) is possible;
Convince people to trust AI systems by telling them which ones they ought to trust (and how much); or
Empower people to make good judgments of an AI system’s trustworthiness when they are considering trusting it for some purpose.
I don’t believe that the first of these is, in general, particularly possible or desirable. The distinction between the second and third is described by Dwyer as trust enforcement vs. trust empowerment.
Empowerment is a human-oriented way to manage questions of trust in AI. If we agree with Gambetta that that trust is choosing to put oneself into a situation of risk, dependent on the actions of another, then the person taking on the risk should be informed and aware of what is being asked of them when they are considering depending on an AI system, and then can choose to engage with it or not on their own terms.
Consider the alternative.
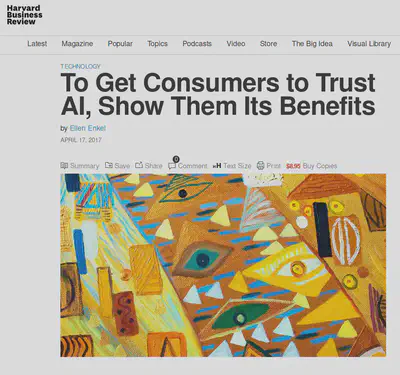
Unfortunately, it is all-too-common these days to read of attempts to ‘get people to trust AI’.
Because, of course, we are building systems that people should trust, and if they don’t it’s their fault, so how can we make them do that, right? Or, we’ve built this amazing “AI” system that can drive your car for you but don’t blame us when it crashes because you should have been paying attention. Or, we built it, sure, but then it learned stuff and it’s not under our control any more— sorry, the world is a complex place.
This isn’t good enough. And at the end of the day, while a company may be able to convince you to use their technology in a risky situation, by clever marketing, one-sided information, or downright deception, this not only means that you have made a poor trust judgment, but that the company was complicit, through intention or ignorance, in making your decision a poor one.
Consider how you’ll feel following a bad outcome, in the cases of trust empowerment and of trust enforcement. If I am convinced or cajoled into relying on a piece of technology, and it fails me, I will typically be annoyed with you that you tried to convince me against my better judgment. If, on the other hand, I have enough unbiased evidence concerning what that system can and will do, and I decide to engage on the basis of that, then I will more likely feel okay afterwards, because I knew what I was getting into.
Can it? Will it?
Evidence of trustworthiness is essential in helping people to make good decisions, in the context of the task, situation, and risk. Perceived trustworthiness is an input into the decision of whether to trust or not, and having good evidence of trustworthiness empowers people to make good trust decisions.
Assessing trustworthiness is broader and more complex than simply determining the probability of success at a task. Trustworthiness judgments are typically based on the competence and character of a potential trustee.
And we can certainly ask questions concerning both the competence and character of AI systems that we and others build, and future ones that might evolve by other means, too.
After all, what could be more relevant or important when considering complex autonomous machines that generate and select behaviours to achieve goals on our behalf in particular contexts?
In AI terms, we can ask of a machine:
Can it do what I need it to?
Will it (decide to) do what I need it to (and when)?
Recent work on trustworthy AI mostly considers the first question. There is a lot of fantastic recent work out there on, to cherry-pick a few examples, runtime verification, safety standards, interpretation of black-box models, resistance of machine learning systems to adversarial attacks, and models that preserve privacy. And we need to draw on this understanding and these techniques.
However, while we have a good and developing grasp on the ‘can it?’ question, surprisingly little research has been conducted on ‘will it?’.
When will an autonomous AI system do what I hope it will do?
We can further unpack this question into concerns relating to the willingness and honestly of the system. Despite sounding anthropomorphic, questions of willingness and honesty in an AI system relate to important factors concerning its goals and capacity to deceive users.
For example:
What runtime goals are present and how do they relate to mine?
Are goals transparent or hidden, does the machine deceive?
When will its goals change?
What is the space of possible goals for the machine?
These are all essential for assessing trustworthiness well.
Final Words
A key aim of my Canada Research Chair program will be to contribute the knowledge and tools needed to provide AI systems with the capability to enhance and expose their trustworthiness to humans.
This inaugural blog post sketched some of the areas we are going to focus on. In a future post, I will talk a little more about how.
Some people reading this will likely be feeling either uneasy or opposed to the idea that trust even has a place at all with human-made machines, and there is certainly a fair amount of recent writing supporting that opinion. The basic argument is that, since AI is a human artefact, humans should be held responsible (or accountable) when it does something ‘wrong’. Well, of course they should. But that doesn’t mean that trust isn’t also in play. And this, too, is something I will come to in future posts.
With thanks for many stimulating conversations, and the borrowing of some passages from co-authored texts, to Jeremy Pitt, Steve Marsh, Simon Powers, and Kirstie Bellman.