Reflective AI
Could computational self-awareness help the trustworthiness of AI?
Professor Margaret Boden has described artificial intelligence as being about making ‘computers that do the sorts of things that minds can do.’ I have always liked this definition, because it does not start from an arbitrary description of the things that might be necessary or sufficient for a system to ‘count’ as AI, but it encourages us to ask: what are the sorts of things that our minds do?
Further, a curious mind is then tempted to ask: could we replicate these things? If so, how? If not, why not?
The definition also implies that there are things that, right now, human minds do, but in the future we might have a computer do instead. This statement is not just true now, but has been at every point in human history, and likely will be far into the future. And it is this transference of activity that gives rise to the seemingly constant stream of examples of new ‘AI technologies’. These mostly do things that human minds used to, or wished to do.
This is, of course, also the source of many of the issues and benefits that arise from the creation and use of AI technology: as we figure out how to replicate some of the things that minds can do, we delegate these things to machines. These are things that human minds would previously have done, or would otherwise have to do, or even in some cases would dream of doing.
This typically brings increased automation, scale, and efficiency, which themselves contain the seeds of both enormous potential social and economic benefit, and potential real danger and strife.
Furthermore, these AI technologies usually do this with an unusual (im)balance of insight and understanding. On the one hand, new, deeper insight and understanding often arise from the models employed, while on the other hand, many of the ‘qualities’ that a human mind would have previously brought to the activity, are utterly absent.
We could cite here many consequences of this delegation phenomenon that can cause concern, such as the rather non-trivial impact of AI on work, or the creation and deployment of autonomous weapons, which are tragically already here. We can also list numerous benefits to humanity and the world; for example this list compiled by Forbes in 2020 includes fantastic improvements in areas from healthcare to wildlife conservation.
Even Chess Isn’t Just About Playing Chess
So what are the sorts of things minds do? As Richard Bellman suggested in 1978, these include ‘activities such as decision-making, problem solving, learning…’ And the sheer quantity of R&D on machines that can do these activities is astounding. Yet as Bellman’s ellipsis suggests, this is clearly an incomplete list. Perhaps any such list would be.
It might be more useful to think situationally. We can ask: which features of my mind do I bring to different activities?
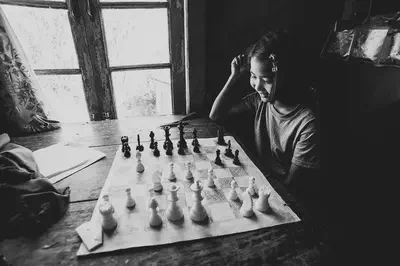
Source: https://globalquiz.org/en/chess-quiz/
Let’s pick a canonical example. If I am playing chess, I largely bring the ability to reason, to plan ahead, to use heuristics, and to remember and recall sequences of moves, such as the caro-kann defence. When I play against an anonymous opponent on the Internet, I generally try to use these abilities as best I can.
When I’m playing chess with my 7 year-old daughter, I bring a few more features too: patience, empathy (I am trying to understand her current mental model of the game to help coach her in what she might want to learn next), and also some compassion, since I could, I suppose, beat her every time and make it less interesting for us both. It’s not that I let her win, that’s not useful either, but I might play out a different sequence of moves to open up more in-game experiences for us from time to time. I have found that this makes it a more interesting and fun thing to do together, and helps both our learning. And as she grows up, benefiting from both more brain development and experience at chess, and finds joy in different parts of the game, the way I do this changes. I find myself thinking back over previous games, and reflecting on the changes in her understanding and reasoning, and responses to my moves. I use this to speculate on and mentally play out possible future games.
This brief journey into the sorts of things my mind is doing when I play chess illustrates three points: first, even playing chess is not just about problem solving; second, rather unsurprisingly, our mental features are rich, contextual, and flexible; and third, we reflect on our situations, our current and past behaviour in them, and the likely outcome of those behaviours including the impact on others, in order to choose which mental features to engage. This is not just about flexible behaviour selection, it’s about which mechanisms – which of Boden’s sorts of things – even kick in.
What can this teach us about how we might want to build AI systems?
Well, returning to the idea that we are delegating mental activity to machines, or at least creating new mental activities that sit alongside our existing ones to support or augment our own work, it tells us that perhaps we ought to have a similar ability in the AI. At least if we are accepting Boden’s invitation to consider which of these sorts of things that minds do we might want.
How like a mind do we want the AI that replaces it to be?
Many people tie themselves in knots trying to define ‘intelligence’, hoping that that will lead us to somewhat of a more complete (and, they often say, more helpful) definition of ‘artificial intelligence’. I think much of this misses the point, at least from the perspective of deciding when we want to accept a computer to replace part of the activity previously done in society by human minds.
Consider: if we are deciding to put a machine into a position where it is carrying out a task, in a way that we are satisfied is equivalent to something that previously only a human mind could do, we have admitted something about the nature of either the task, or the machine, or our minds.
Perhaps an AI system is simply a machine that operates sufficiently similarly to our mind, at least in some situation, that we are prepared to accept the machine operating in lieu of it.
So this leads us to ask when and why we would be prepared to accept this. Or perhaps, given most AI systems (and minds) cannot be not fully understood or controlled, when and why we would be prepared to trust it to do so.
Just Bad Apples?
Let’s pick another now well-known example: Amazon’s automated recruiting tool, trained on data from previous hiring decisions, that discriminated based on gender for technical jobs. Here, the delegation is from recruiters and hiring managers to a computer that replicates the mental activity they used to do. The aims are automation, scale, and efficiency.
Are cases like this just bad apples?
That such a sexist system was put into practice at all is at the very least unfortunate and negligent. But what is particularly interesting in the context of the discussion in this article is that, not only did the mind-replacing-AI not know that it actions were contra a strong social norm (or even a law), but that it did not even have the mental machinery to know such a thing. It had no way of noticing that this had happened, and reflecting on this, in order to be able to stand a chance at correcting itself (or simply stopping in the knowledge that it was doing harm). This part of the mental activity is, as yet, nowhere near delegated. This leads to an unusual divorce of accompanying mental qualities that would normally work in concert. No wonder the behaviour might seem a little pathological.
In this situation, people – hiring managers, shareholders, applicants, and others – trusted the system to do something that, previously, a human mind did. But unlike the mind of the professional it replaced, it had no way of reflecting on the social or ethical consequences, or on the virtue or social value of its actions, or even if its actions were congruent with prevailing norms or laws.
As humans, we reflect in these ways. Reflection is a core mental mechanism that we use to evaluate ourselves. The existence of this form of self-awareness and self-regulation can be key to why others may find us trustworthy. Could we expect the same of machines?
What Should We Expect AI To Do?
Remaining at the level of intuitive, natural statements around requirements of AI systems (which is where, I believe, most people operate), we might consider that the typical expected scope of AI activity has broadened substantially. Consider the progression through the following sorts of ‘requirements statements’:
- Play chequers convincingly.
- Beat a grandmaster at chess.
- Distinguish between malignant or benign lesions from ultrasound data.
- Play the game Starcraft at approximately human level.
- Drive a car on a sunny highway.
- Drive a car through the middle of a city, in the rain, past a busy school at home-time.
- Select some promising candidates from this large pool of CVs, given a job specification.
- Have helpful conversations with my customers through a chat window.
All of these are, to varying degrees, technologically challenging. And the latter examples have substantially more complex situational detail and nuance. How ought a machine do that?
Of course, these vague requirements must be fleshed out and probably quantified before an implementation can be attempted. But that does not necessarily change the nature of the intuitive statement at the root. In the work I’ve done with organisations, applying AI in their workflows for the first time, I have found there can often be a general expectation that this translation is ’technical work’ and that there is trust that the experts will faithfully re-represent their intention into the requirements of the machine. And that’s actually okay: most people are not experts in what to expect from the AI du jour, nor should we expect them to be. We should no-more expect or require the people who work with AI alongside them to be experts in AI, as I would expect to understand how a clutch works — or even know if my car has one — in order to drive.
This post is not the place to get into the nitty-gritty of what the requirements for a CV-filtering agent should contain, but it is hopefully immediately obvious that it should not do anything illegal: for example, start filtering based on gender, or trans status, or age, or ethnicity. Nor, I might suggest, would it be right for an AI system to encode a rule that says ‘you went to X university and typically people from there don’t do so well with us, so we will rule you out’. This may have been a lazy shortcut made by some human recruiters, but with machines that can’t be lazy, we ought to expect that we can do better.
Socrates’s Daemon
So how do we know when we’ve done something, or are about to do something we ought not? Well there are different approaches we can take, here. One involves capturing all these issues as requirements, and representing them as constraints in the model. We can use things like penalty functions to encourage the learning of solutions that don’t do this. Taking this approach is a little like creating a perfectly virtuous mind that is incapable of ill thought. A lovely ideal, maybe, but perhaps somewhat creatively constraining.
An alternative approach is to be reflective. To have perhaps what Socrates called his daemon, something that , checked him ‘from any act opposed to his true moral and intellectual interests.’
Socrates saw this check as a divine signal, something that did not propose action, but monitored it, and intervened if necessary. If such a check were based on morals or ethics, we might call this a conscience. If it were based on broader goals than simply the immediate (for example, choosing a chess move to make against my daughter), we might call this considering the bigger picture.
Essentially, this is a process that notices what we are thinking, what we are considering doing, and allows the thought, but can prevent the action. It decides whether to do this based on contextualising the action, and possibly exploring its consequences. Contexts, as alluded to above, might be ethical, cultural, political, social, or simply based on non-immediate (higher-level, longer-term, or not immediately visible) goals.
The advantage to the reflective approach is that it is a separate process, and we know that building systems that monitor themselves is usually easier using an ’external layer’ architectural approach. In this case, I don’t need to re-learn my decision model if something in the set of oughts in my situation changes (though I might want to, later). I just need to check the behaviour against them, and occasionally say ’no, that’s not appropriate; give me an alternative, try a different approach.’
Consider again chess: I don’t need a different model of how to play the game for each different situation: I can use a model I learnt playing strangers better than me online, when playing with my daughter. I just need that model to provide a variety of possible options, and for me to have a reflective mechanism for choosing between them in context.
We can say the same of a CV filter system. When I am trying to filter a list of hundreds of applications for the role of female caregiver for vulnerable adults, where being female is an important and legally-permitted characteristic of the job, it is super-helpful to have a rule that filters out all the men and only shows me the women. When I’m trying to recruit trainee programmers, I want the machine to actively ignore or even identify and correct the very likely bias that will be in its training dataset. But both are within the range of permissible thought for the mind-like thing. We just need to know which is the right or wrong one to do in the context.
Reflection and AI as a Professional Practitioner?
Reflection can go deeper still. As a teacher and in the leadership roles I have held, I use reflective practice a lot. I practice it myself, reflecting for example on lectures I’ve given and interactions with colleagues. I often spend time with students, helping them to develop their reflective practice, hopefully empowering them to be their best selves, exactly by learning to reflect. Reflective practice has its origins in many ancient teachings, for example in Buddhism and indigenous knowledge.
Donald Schön, in his book The Reflective Practitioner: How Professionals Think in Action, considers the inherent nature of reflection in professional practice, comparing it with a purely technically rational approach that might be characterised by up-front specification and subsequent problem solving:
‘From the perspective of Technical Rationality, professional practice is a process of problem solving. Problems of choice or decision are solved through the selection, from available means, of the one best suited to established ends. But with this emphasis on problem solving, we ignore problem setting, the process by which we define the decision to be made, the ends to be achieved, the means which may be chosen. In real-world practice, problems do not present themselves to the practitioner as givens. They must be constructed from the materials of problematic situations which are puzzling, troubling, and uncertain.’
This means that, the sorts of things that (to continue the example) a professional recruiter does is not simply problem solving in a defined setting. There are patterns and mechanical aspects to their work, but the problem is always somewhat uncertain, and emerges from practice and the setting. By reflecting on these, argues Schön, the problem becomes more apparent. This is inherently ongoing, incomplete, and messy.
For example, it may not have been apparent that a particular aspect of the practice was a necessary part of the problem specification, until it emerged, and then the professional was required to ’think on their feet’. Requirements are never complete until we have considered ‘all realizable classes of input data in all realizable classes of situations’, and it is not hard to see that an open-ended socio-technical context makes this rather challenging, even in seemingly narrow domains like CV filtering.
Observing professionals and experts in walks of life from medicine to baseball, Schön proposes the need to understanding how they think and act using ‘an epistemology of practice which places technical problem solving within a broader context of reflective inquiry.’
To pick an example that I imagine is familiar to many readers, for me reflective enquiry is perhaps the single most useful skill in being a good programmer. It is a way of formalising and accepting the uncertainty, confusion, uncomfortableness, and curiosity, that we must deal with when ‘construct[ing the problem] from the materials of problematic situations which are puzzling, troubling, and uncertain’ (to recall the quote).
Reflection of this sort enables us to learn from doing, updating our knowledge and understanding of the problem and how to solve it as we go, and to fail forward.
Schön’s book is worth reading in its entirety, especially if we deeply want to understand what sorts of mental things might need to be involved in delegating professional activities to machines in a way that we really are more inclined to consider equivalent.
But an obvious question is how do we do reflection?
How Do We Do Reflection?
Another two modern pioneers in this area, David Kolb and Ron Fry, developed a model that captures the role of reflection in practice that is both exploratory and governed by a sense of the bigger picture and the principles we hold that govern our intended direction.
Their ‘Experiential Learning Model’ comprises four phases, arranged in a cycle:
- having a concrete experience,
- an observation and subjective evaluation of that experience,
- the formation of abstract concepts based upon evaluation,
- the formation of an intention to test the new concepts, leading to further experience.
This cycle then repeats.
This mode was subsequently developed further by Kolb, such that it is now often referred to as Kolb’s learning cycle.
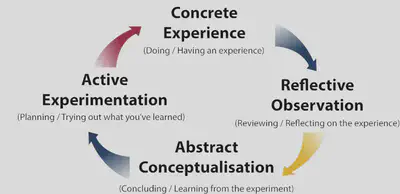
Source: https://www.queensu.ca/experientiallearninghub/about/what-experiential-learning
A more elaborate way of thinking about and capturing the outcome of this reflective process might look something like the following:
What happened? This leads to a statement of facts concerning a situation I was involved in.
What was it like? This requires a statement of judgment concerning those facts. Here we compare the result of the experience against the values or expectations captured for example in our conscience, bigger-picture expectations, higher-level goals, or reactions of ourself or others. It gives us the beginnings of an awareness concerning how we relate to the specifics of the experience.
What have I learnt about myself? This should give us a new or updated self-model, based on integrating the judgments into our existing knowledge and understanding. Here we conceptualise our newly emerging self-awareness.
What should or shouldn’t I do in the future, or what might I try? This allows us to update our behaviour regulator, our Socrates’s Daemon, based on our new self-knowledge. It can provide new behaviour restrictions, or new permissible avenues to explore.
At this stage, some may be wincing at the idea of a machine ’experiencing’ something akin to ‘what it’s like’ to do X. But here’s the thing: this is the language of the mental ’thing’ we are exploring replicating; we do not need to take a philosophical position anywhere near the idea that machines can have qualitative experience in the same way that we do. We merely need to admit that something that fulfils the same role can be produced, here, that they can evaluate an experience from their own perspective on the world (i.e. given their own sensing and self-monitoring machinery). And there are plenty of ways of doing that, from simple familiar things like evaluation functions to more nuanced models of emotion or affect and self-modelling.
In fact, I have come to the view that not understanding what we are doing with the language at this stage of the process of conceptualising mind-like machines is often the source of much hype, misunderstanding, and denial. But that is for another post!
Others may be looking at the description of the reflective cycle above and be thinking that it looks pretty much like like reinforcement learning. And they wouldn’t be wrong: many researchers have been using reinforcement learning for machine self-awareness, in specific ways, for several years. The trick is perhaps not in the architecture of the cycle, or some fundamentally new algorithm, but in the nuance of application: the machine directing the observations and judgments toward itself at the meta-level; making those judgments concerning higher-level sets of expectations (emotional responses, norms, social values, ethics, laws) rather than those that are immediately obvious from a specification of the task at hand; and explicitly modelling itself in relationship with the world when doing this.
There are many models of reflection that we can draw on – Kolb’s is but one. And they all largely follow the same architectural pattern, and broadly speaking, exist for the same purpose. Which to use is perhaps less important than the idea that we can use them in this way.
A Bridge Too Far?
Let us return to the idea of flexible mental mechanisms of different sorts, accompanied by reflective processes to help decide when to deploy them.
Perhaps one reason why we rarely came across the need for these sorts of features of AI in years gone by is that the sorts of delegations to machines were highly restricted and well-scoped. Deep Blue was designed to optimise the game of chess, within its operating constraints; it was not designed to provide entertaining or interesting games, nor to empathise with learners of the game. Nevertheless, it is clear that interesting and educational things can fall even out of this approach, as evidenced by AlphaGo’s Move 37 – though only interesting to us, and only if we took the time to notice and reflect.
It is also worth noticing that even the most ardent skeptics of AI may not disagree. For example, James Lighthill in his now infamous report that virtually shut down AI research in the UK in the 1970s, and is largely credited with triggering the first ‘AI Winter’, noted that:
‘Most robots are designed from the outset to operate in a world as like as possible to the conventional child’s world as seen by a man; they play games, they do puzzles, they build towers of bricks, they recognise pictures in drawing-books; although the rich emotional character of the child’s world is totally absent.’
Could it be that AI skeptics such as Lighthill appeared as such, simply because they saw the mind as a richer thing than a solver of games? Lighthill also noted that the majority of AI research at the time was being carried out by men (spoiler: it still is). And, I think it fair to say, he saw an unhealthy bias in the discipline towards building robots that, frankly speaking, were designed to play with boys’ toys.
Perhaps we have no business expecting or targeting the creation of machines that can do the sorts of things I discussed in this post that a human mind does, and stay within the domain of well-specified problem solving. In this way, it is clear where the dividing line is between the machine (that is simply executing the instructions it was given) and the people who are responsible and accountable for its actions. Then again, perhaps this isn’t always going to be as possible as we’d like; we might need to moderate our expectations, then.
Building Reflective AI
But if we did want to explore this possibility, what would we need?
Well, first let’s get our house in order. When building and deploying AI systems, most of us could certainly be better at making them context-sensitive. We ought to better understand stakeholders and operational conditions, do better requirements analysis, spend more time on deeper analyses of bias in data, be more transparent about training sets, and expect more interpretable decision models. What I am proposing here is not an either-or.
But, can a machine be reflective, too? In the ways that humans are, and try to be? Can we expand our list of Boden’s ‘sorts of things’ to include this sort of reflection? Can we literally give AI a conscience?
What would be needed?
First we need the architecture. We must separate out reflection from decision making and action.
Second, we need decision models to not just propose a single ‘optimal’ solution, but be ready to present a diversity of possible ways forward, based on different approaches to solving the problem (including safe ways of disengaging from it).
Third, we need a reflective process able evaluate proposed decisions in context (acknowledging that context can change) and at least block some actions and – even better – feed back to the decision model saying what about it was not appropriate, to inform learning.
Finally, we need a way of representing the broader context: we need models of prevailing norms, and of the social values associated with the outcomes of different possible actions, and of other higher-level goals that may not be immediately or obviously relevant to the task.
Thankfully, there is already a lot of work that we can lean on, to help us to do this:
There is now several decades of work on reflective architectures, including early influential work like Landauer and Bellman’s Wrappings, and those such as the EPiCS architecture, originally developed for use in a large EU research project, and the LRA-M architecture that emerged from a collective effort at a 2015 Dagstuhl seminar, both aiming explicitly at achieving computational self-awareness through reflection. It is likely that new and extended ways of architecting reflective systems can also be developed, based on the discussions about reflection, above. We also have more than a decade of work on techniques for self-modelling in complex system engineering, and examples of self-simulation in domains as disparate as robotics and service-oriented computing.
Explicitly ethical agents are nothing new, either. Even leaving aside explorations in science fiction and literature, for a moment, we have been seriously thinking about ethical or moral machines at least since Moor proposed a way of discerning four different ’types’. Indeed, the question of how to imbue artificial agents with ethical values was the topic of a special issue of Proceedings of the IEEE, already two years old. Winfield et al’s summary provides an introduction to the topic, and some of the challenges involved. Cervantes et al’s survey dates from around the same time. And indeed, there is some great early work from Alan Winfield’s lab, on putting these kinds of ’ethical governors’ into robots. Alan has also raised some serious concerns about whether explicit ethical agents are a good idea. The explorations continue.
We also need to respond to our ‘conscience’ from time to time by finding alternative ways of approaching problems. When considering finding multiple possible diverse and viable courses of action, we can draw on the rich and active research activity on so-called Quality Diversity Algorithms. Related techniques like Novelty Search could help us to find new, different solutions, that come at a problem from a novel angle. And when evaluating these alternatives, we may choose to formulate the very notion of what ‘successful’ means according to our values; techniques from game theory, such as solution concepts may provide us with the necessary tools, and in adopting these we must acknowledge that the best action may be a compromise.
Anyone who has studied philosophy will tell you that the formalisation of and reasoning with norms is a well established field. There is a fascinating intersection of philosophy, law, mathematics, and computer science, studying deontic logic – the logic of obligations and permissions – itself just a few years shy of turning 100. Of a more practical nature, research on capturing norms in human-robot interactions is also alive and well. Clearly, an AI system able to reflect on its actions in terms of social norms would need to draw on formal models such as these.
So, there’s lots out there! And also a lot of work to do in understanding how to put together the pieces of the puzzle.
A Way Forward?
Often, I find the most interesting work in artificial intelligence is not breaking a problem down until its constituent parts are solvable (though this is clearly important work), but in the linking of these things back together again, to create the sorts of complex mind-like phenomena that motivated us in the first place. In this post, I have tried to sketch some thoughts regarding one area in which it might be valuable to do this.
As a final word, I want to ask if we are falling into the trap of trying to produce what Max Oelschlaeger called ’the myth of the technological fix’? In other words, are we trying to provide a technological solution to what is fundamentally a social problem? This would clearly be an issue, but I don’t think so. What I am proposing is a socio-technical mechanism for providing social solutions to social problems, in the context of AI technology. After all, to use an analogy, libraries are simply buildings, paper, and databases, that are built by people and enable us to enlighten, inform, and provide pleasure to the population at large. Reflective AI could be a set of methods, tools, and technologies that enable us to contextualise, socialise, put sensitivity into, enrich, and build trust with artificial intelligence technology.